Categories : Python
Yolov8 이미지라벨링
Yolov8 이미지라벨링 설명
0. Yolov8 소개
YOLOv8(You Only Look Once version 8)는 Ultralytics에서 개발한 객체 탐지(Object Detection) 및 분할(Segmentation) 알고리즘 YOLO 시리즈는 실시간 객체 탐지에서 높은 성능과 속도가 장점임 활용분야로는 자율주행, 보안, 의료, 소매업, 로봇공학에 사용된다.
1. Python 패키지 설치 및 세팅
python venv [folder] # 가상환경 세팅
pip install ultralytics opencv-python labelme2yolo numpy
2. 라벨링 프로그램 다운로드
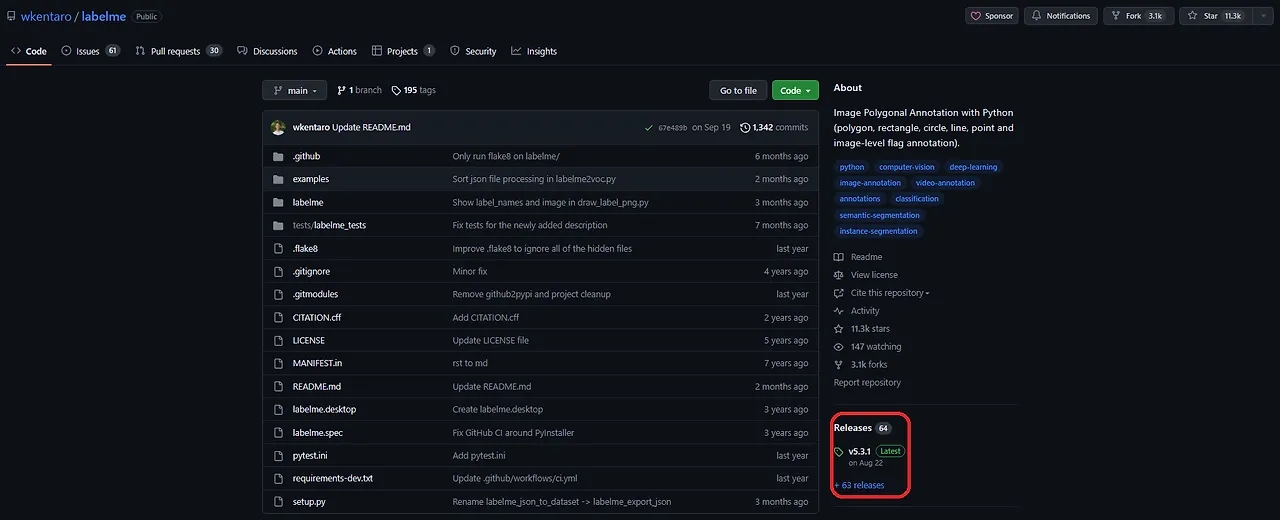
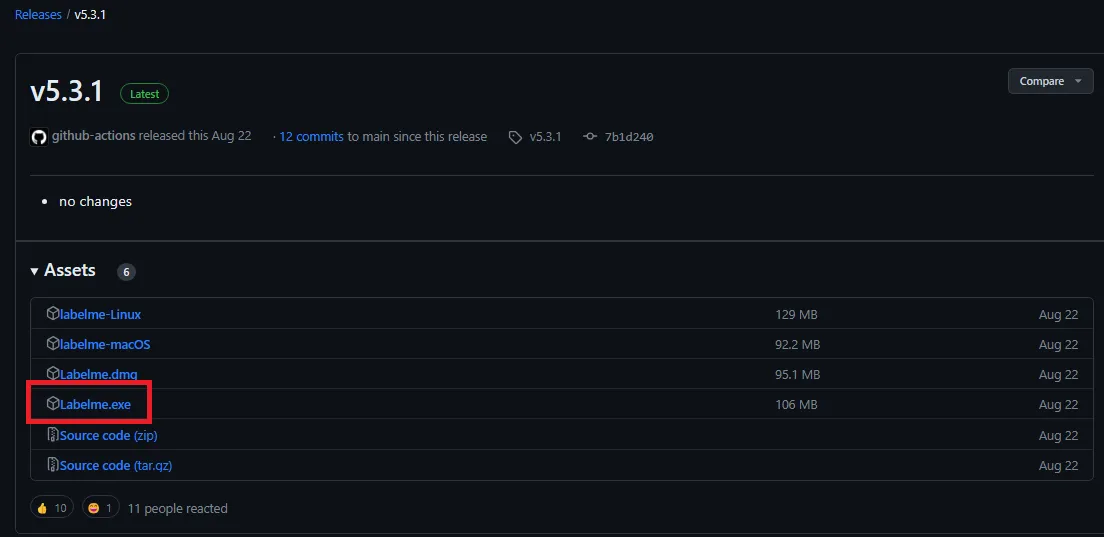
3. 라벨링 실행
Open Dir -> 학습할 이미지가 있는 폴더
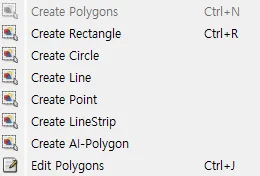
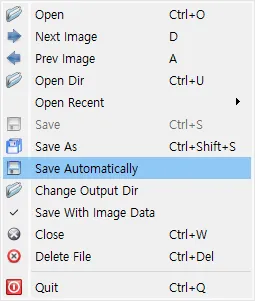
A : 이전이미지
D : 다음이미지
4. 라벨링 결과 확인
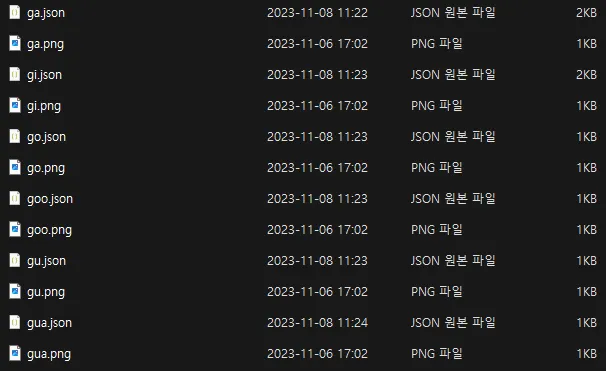
json과 함께 같이 저장됨
5. 라벨링 데이터셋 만들기
labelme2yolo --json_dir [라벨링 저장된 json파일있는 폴더] --val_size 0.15 --test_size 0.15
라벨링 저장된 폴더에 dataset.yaml이 저장됨
해당 단계에서 만들어진 폴더를 다른곳으로 옮길때는 ‘dataset.yaml’ 파일을 열었을대 파일경로데이터를 수정해줘야한다
train, val, test
6. 학습시키기
yolo detect train data=[dataset.yaml파일위치 .yaml까지] model=yolov8n.pt epochs=100 imgsz=640`
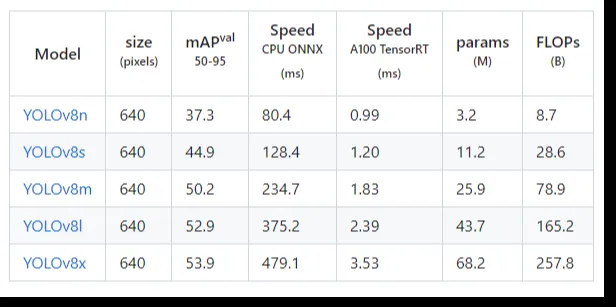
n 에서 x로 갈수록 학습 속도 느려짐
학습완료되면
runs > detect > train > weights > best.pt 로 저장됨
7. pt 활용 방법 예시
from ultralytics import YOLO
model = YOLO("yolov8s.pt") # 방금 학습한 pt로 바꾸면됨
results = model("https://ultralytics.com/images/bus.jpg", save = True) # 가져와볼 이미지, 비디오 / save =True 해야 저장, show = True 해야 보여줌
# print(results)
# 결과
image 1/1 C:\Users\xxxx\Desktop\yolovenv\bus.jpg: 640x480 4 persons, 1 bus, 468.4ms
Speed: 32.2ms preprocess, 468.4ms inference, 2.0ms postprocess per image at shape (1, 3, 640, 480)
or
from ultralytics import YOLO
model = YOLO("yolov8s.pt")
results = model("https://ultralytics.com/images/bus.jpg", save = True)
for result in results:
### xyxy
print(result.boxes.xyxy)
### conf
print(result.boxes.conf)
### cls
print(result.boxes.cls)
### id
print(result.boxes.id)
### xywh
print(result.boxes.xywh)
### xyxyn
print(result.boxes.xyxyn)
### xywhn
print(result.boxes.xywhn)
# 결과
Found https://ultralytics.com/images/bus.jpg locally at bus.jpg
image 1/1 C:\Users\xxxx\Desktop\yolovenv\bus.jpg: 640x480 4 persons, 1 bus, 633.7ms
Speed: 9.5ms preprocess, 633.7ms inference, 3.0ms postprocess per image at shape (1, 3, 640, 480)
tensor([[1.9989e+01, 2.2960e+02, 8.0562e+02, 7.4782e+02],
[6.6772e+02, 3.8936e+02, 8.0967e+02, 8.7938e+02],
[5.0511e+01, 4.0051e+02, 2.4500e+02, 9.0293e+02],
[2.2200e+02, 4.0819e+02, 3.4526e+02, 8.6076e+02],
[1.7374e-01, 5.5013e+02, 6.7284e+01, 8.6807e+02]])
tensor([0.9202, 0.8988, 0.8873, 0.8805, 0.6382])
tensor([5., 0., 0., 0., 0.])
None
tensor([[412.8051, 488.7100, 785.6316, 518.2142],
[738.6946, 634.3691, 141.9548, 490.0229],
[147.7544, 651.7199, 194.4868, 502.4294],
[283.6280, 634.4742, 123.2612, 452.5717],
[ 33.7287, 709.0996, 67.1100, 317.9405]])
tensor([[2.4678e-02, 2.1260e-01, 9.9459e-01, 6.9242e-01],
[8.2434e-01, 3.6052e-01, 9.9959e-01, 8.1424e-01],
[6.2359e-02, 3.7084e-01, 3.0247e-01, 8.3605e-01],
[2.7407e-01, 3.7795e-01, 4.2625e-01, 7.9700e-01],
[2.1450e-04, 5.0938e-01, 8.3066e-02, 8.0377e-01]])
tensor([[0.5096, 0.4525, 0.9699, 0.4798],
[0.9120, 0.5874, 0.1753, 0.4537],
[0.1824, 0.6034, 0.2401, 0.4652],
[0.3502, 0.5875, 0.1522, 0.4190],
[0.0416, 0.6566, 0.0829, 0.2944]])
or
from ultralytics import YOLO
import ast
model = YOLO("yolov8s.pt") # 방금 학습한 pt로 바꾸면됨
results = model("https://ultralytics.com/images/bus.jpg", save = True) # 가져와볼 이미지, 비디오
data = {0: 'person', 1: 'bicycle', 2: 'car', 3: 'motorcycle', 4: 'airplane', 5: 'bus', 6: 'train', 7: 'truck', 8: 'boat', 9: 'traffic light', 10: 'fire hydrant', 11: 'stop sign', 12: 'parking meter', 13: 'bench', 14: 'bird', 15: 'cat', 16: 'dog', 17: 'horse', 18: 'sheep', 19: 'cow', 20: 'elephant', 21: 'bear', 22: 'zebra', 23: 'giraffe', 24: 'backpack', 25: 'umbrella', 26: 'handbag', 27: 'tie', 28: 'suitcase', 29: 'frisbee', 30: 'skis', 31: 'snowboard', 32: 'sports ball', 33: 'kite', 34: 'baseball bat', 35: 'baseball glove', 36: 'skateboard', 37: 'surfboard', 38: 'tennis racket', 39: 'bottle', 40: 'wine glass', 41: 'cup', 42: 'fork', 43: 'knife', 44: 'spoon', 45: 'bowl', 46: 'banana', 47: 'apple', 48: 'sandwich', 49: 'orange', 50: 'broccoli', 51: 'carrot', 52: 'hot dog', 53: 'pizza', 54: 'donut', 55: 'cake', 56: 'chair', 57: 'couch', 58: 'potted plant', 59: 'bed', 60: 'dining table', 61: 'toilet', 62: 'tv', 63: 'laptop', 64: 'mouse', 65: 'remote', 66: 'keyboard', 67: 'cell phone', 68: 'microwave', 69: 'oven', 70: 'toaster', 71: 'sink', 72: 'refrigerator', 73: 'book', 74: 'clock', 75: 'vase', 76: 'scissors', 77: 'teddy bear', 78: 'hair drier', 79: 'toothbrush'}
for result in results:
numberid = str(result.boxes.cls).replace('tensor(', '').replace(')','')
rr = ast.literal_eval(numberid)
convert = [data[int(item)] for item in rr]
print(convert)
# 결과
['bus', 'person', 'person', 'person', 'person']
8. 참고자료
9. pt 활용 하면서 생긴 것들
labelme2yolo --json_dir C:\Users\aaaa\Desktop\images --val_size 0.15 --test_size 0.15 yaml만들기
yolo detect train data=C:\Users\aaaa\Desktop\YOLODataset\dataset.yaml model=yolov8n.pt epochs=100 imgsz=640
yolo detect predict model=C:\Users\aaaa\Desktop\yolovenv\runs\detect\train\weights\best.pt source=[적용해볼 동영상 파일경로]
yolo predict model=C:\Users\aaaa\Desktop\yolovenv\runs\detect\train\weights\best.pt source='gogogo.JPG'
pip install torch==1.7.0+cu92 torchvision==0.8.1+cu92 -f https://download.pytorch.org/whl/torch_stable.html